Augmented Analytics: Revolutionizing Data Insights for Smarter Business Decisions
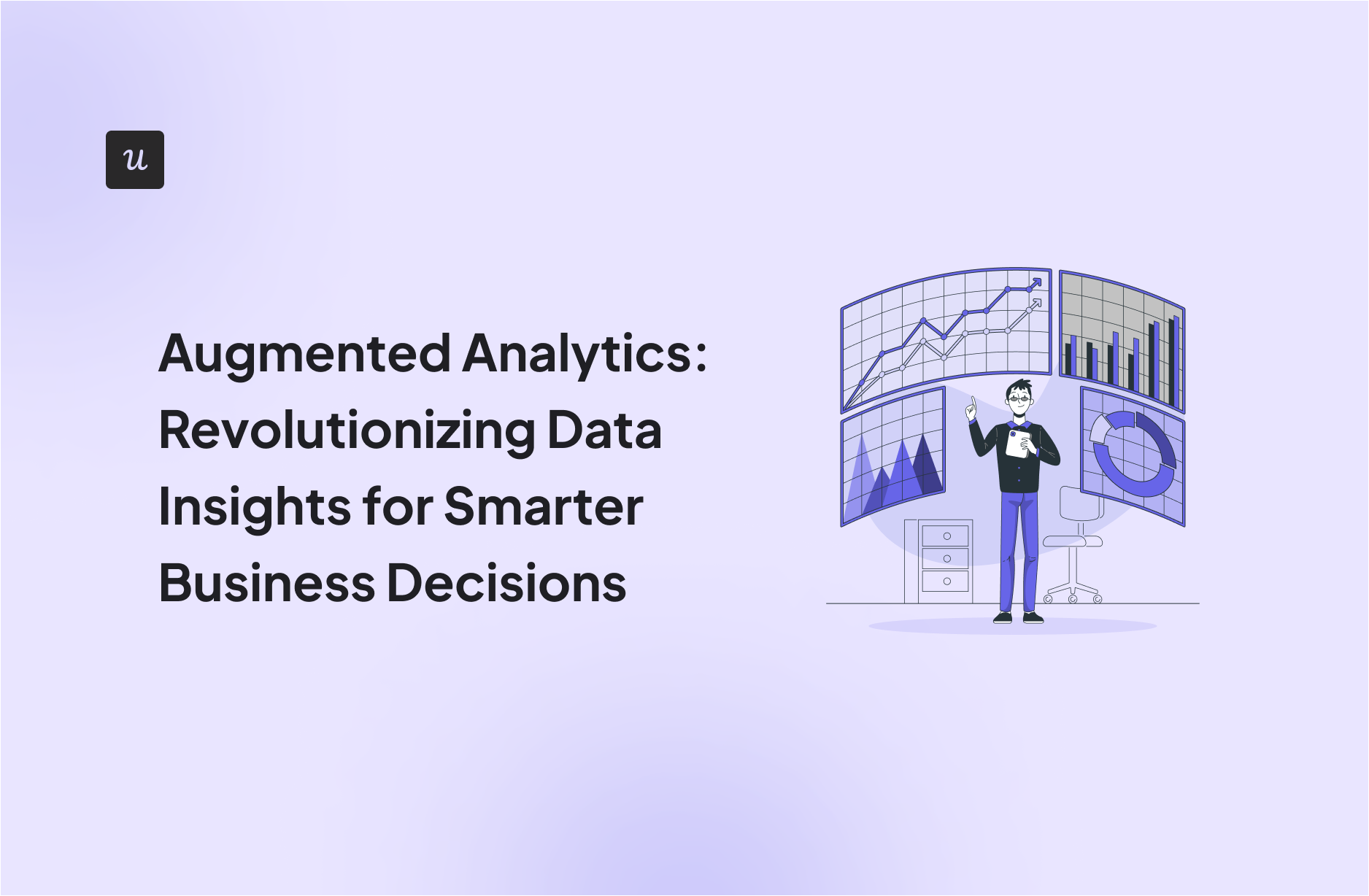
What is augmented analytics?
Augmented analytics represents a sophisticated approach to data analytics, leveraging the capabilities of artificial intelligence (AI) and machine learning (ML). It elevates our interaction with data by providing contextual understanding, thereby streamlining and simplifying the process of data analysis.
Imagine possessing an intelligent ally that not only comprehends your datasets, but also proffers insights and recommendations to bolster your decision-making processes. This is essentially what augmented analytics provides.
Augmented analytics tools play a pivotal role in this evolution, automating data preparation, simplifying integration with various data sources, and enhancing insight discovery through advanced techniques like natural language queries and machine learning algorithms.
What truly revolutionizes the field is augmented analytics’ ability to make data analysis widely accessible across an organization irrespective of individual technical expertise, thus broadening its utilization. So whether one is a corporate leader attempting to navigate market trends or you’re among those in the role of data analysts seeking more nuanced analyses, augmented analytics stands ready as an inclusive solution for various levels within an entity.
The role of AI and machine learning in augmented analytics
AI and machine learning are substantial drivers of augmented analytics, revolutionizing data analysis by automating processes, customizing insights, and simplifying the steps involved in preparing data.
Through leveraging machine learning algorithms to analyze vast quantities of data, they detect trends, irregularities, and links that contribute to the development of sophisticated data models. These algorithms are particularly adept at helping to identify patterns, which is crucial for predicting future trends and making data-driven decisions.
Imagine a system capable of integrating your company’s semantics while adapting to user preferences and independently generating insights—this is what machine learning contributes. This technology not only accelerates the pace at which analyses are performed but also enhances access to data analytics for a broader range of users. In turn, this democratization allows more individuals within an organization to make decisions based on solid evidence drawn from their available information sources.
Natural language processing for insight generation
Have you ever wished for a clear answer to your questions about data? Natural language processing (NLP) coupled with natural language generation can make this wish come true! NLP allows us to use simple linguistic queries when interacting with data, making it easier to overcome the traditional hurdles of querying and unlock insights in an uncomplicated manner.
Meanwhile – tools like Userpilot offer easy-to-use dashboards that allows you to track key metrics at a glance – and soon also query them with natural language:
NLP doesn’t just facilitate our questioning. It also translates intricate data and analytical results into narrative explanations and summaries. This capability helps in pinpointing trends within the information. Understanding insights from complex datasets is made possible even for those without deep expertise in the field, benefitting not only lay users but seasoned data scientists too.
This advancement leads us towards a more nuanced and dialogical way of engaging with datasets. It delivers findings that are significantly more digestible by laying them out through intuitive narratives.
Advantages and challenges of adopting augmented analytics
Augmented analytics comes with several benefits that are instrumental in enhancing data tasks:
- It expedites tasks associated with data.
- It accommodates queries formulated in natural language.
- Without prompt, it provides insight recommendations.
- It enables quicker decision-making processes.
- By making data accessible to all, it cultivates a culture driven by data within companies. Additionally, augmented analytics facilitates data discovery, a crucial process that saves time and energy in data exploration and analysis through the use of augmented analytics and artificial intelligence.
At present, one-third of organizations have embraced augmented analytics. They’re reaping its rewards and tackling the accompanying challenges head-on for their business users.
This journey is not without hurdles. The widespread adoption of augmented analytics faces various trials that necessitate deliberate consideration and solutions. Such obstacles include issues around the accuracy of insights provided, relevance to specific contexts and potential biases inherent in the underlying datasets which could compromise the integrity of generated insights.
Benefits of augmented analytics
Augmented analytics provides a multitude of benefits, streamlining the process of data analysis for those without technical expertise and thereby making insights from data accessible to all. This contributes to fostering an environment where decisions are informed by data across the entire organization.
Entities employing augmented analytics reap several rewards.
- Acceleration in the readiness of data for use.
- Attainment of more profound understandings.
- Enhanced perception of data through highlighting interconnections, patterns, and anomalies within datasets.
- Economic advantages stemming from decreased need for technical assistance and prevention of unnecessary duplication of data.
Challenges in implementing augmented analytics
Despite the significant advantages of augmented analytics, it’s equally important to be aware of the accompanying challenges. Issues of accuracy, relevance, and data biases can impact the quality of insights. Overcoming these requires robust data management processes and a good understanding of the nuances of data analysis. To effectively analyze data, one must be mindful of these challenges and take necessary precautions.
Another hurdle is scaling up augmented analytics systems to handle extensive data volumes. This requires significant computing power and can pose a challenge for some organizations. Additionally, businesses face the task of dispelling misconceptions about augmented analytics and fostering confidence in the technology for successful adoption and integration into operational processes.
Real-world applications of augmented analytics
Augmented analytics have been instrumental in elevating the understanding of customer dynamics, optimizing business operations, and bolstering decision-making across various sectors. Within retail, this advanced analytical approach drives strategic business decisions by examining patterns in customer behavior to enhance marketing efforts and manage inventory more effectively.
Similarly, healthcare entities use augmented analytics for superior patient services, anticipating disease spread, and making clinical practices more efficient. Sports organizations, manufacturers, as well as those within media and entertainment are harnessing the power of augmented analytics to boost performance metrics, operational efficacy, and deepen consumer interaction.
The widespread integration of these sophisticated analytical tools demonstrates their pivotal role in informing smarter business strategies across different fields. Augmented analytics tools aid in data discovery and help businesses identify patterns in customer behavior, operational data, etc., streamlining the process of making data-driven decisions.
Key components of augmented analytics platforms
At the heart of augmented analytics platforms lie AI and machine learning, which are crucial for enhancing data understanding, analysis, and the generation of insights. These technologies automate essential operations, including data management, analytical modeling, and discovery tasks, lessening the need for expert handling in these areas. With advanced analytics tools at their disposal, companies are poised to sift through their own information troves more effectively to make knowledgeable choices.
The advantages offered by such platforms include:
- The facilitation of user engagement with datasets via voice commands that streamline analysis.
- Utilization of natural language processing which renders data examination more instinctive.
- Automated identification of optimal datatypes for use in predictive models.
- Consistent surveillance over model efficacy.
Equipped with these capabilities, analytics platforms stand out as indispensable tools for data analysis.
Userpilot: Enhancing product analytics with augmented analytics
Leveraging the power of augmented analytics, Userpilot emerges as a comprehensive platform designed specifically for product teams. It encompasses features such as in-app engagement, detailed product analytics, and integrated in-app surveys.
Userpilot sets itself apart by offering advanced analytical features. It excels in identifying unusual data patterns, providing users with timely notifications about these occurrences, and offering insights into future trends. With tools for anomaly detection, root cause analysis, and trend forecasting, the platform significantly boosts its product analytics capabilities – positioning Userpilot as a valuable tool for businesses.
Best practices for implementing augmented analytics
The effective deployment of augmented analytics requires careful planning and tactical execution. This begins with the selection of an appropriate platform that supports deployments in cloud-based and on-premises environments, reinforced by strong cybersecurity measures.
Organizations must automate their data management procedures to amalgamate various data sources like business data into a single stream, convert this information into a standardized format, and subsequently store it within data warehouses.
There’s a need for organizations to comply with industry standards regarding quality regulation in data management and adhere to legislation pertaining to the protection of such data. It is also crucial for businesses to give precedence to safeguarding their data assets while simultaneously fostering training programs aimed at enhancing skills related not only to general understanding but also specifically focused on analytical prowess within the sphere of data science.
Conclusion
Augmented analytics is revolutionizing various industries by enhancing decision-making and improving customer experiences with insights powered by artificial intelligence. This innovation boosts productivity and speeds up the decision process through automation of tasks such as data preparation and identifying patterns, thanks to augmented analytics capabilities.
As it makes data analysis more accessible while offering predictive and prescriptive insights, augmented analytics promises to transform business decision processes. We can expect future developments in this technology to bring about even more sophisticated AI models along with improved automation features in analytical tools.
Frequently asked questions
What is augmented analytics?
Augmented analytics represents a sophisticated variant of data analysis, leveraging AI and machine learning to amplify the process of analyzing data. This approach broadens the accessibility of data analytics to individuals across varied levels of technical expertise, thereby democratizing the extraction of insights from data for a wider audience.
How does AI and machine learning contribute to augmented analytics?
Machine learning, a subset of AI, enhances augmented analytics by automating operations and refining the handling of data. It also becomes attuned to the semantics within an organization and evolves in response to individual user inclinations, delivering tailored insights.
As a result, analytics become both more effective and customized for users.
What are the benefits of augmented analytics?
Enhanced by augmented analytics, non-technical users can achieve accelerated data preparation and simplified analysis, while gaining more profound insights and reducing costs.
What challenges are faced in implementing augmented analytics?
Successful implementation of augmented analytics demands overcoming obstacles related to precision, relevance, biases within the data, and the capacity to scale systems effectively for extensive data quantities. Addressing these challenges is crucial for effective deployment.
How is Userpilot enhancing product analytics with augmented analytics?
Utilizing artificial intelligence, Userpilot bolsters product analytics through the detection of anomalies and trend forecasting while promptly notifying users. This advancement aids in pinpointing the fundamental reasons behind these anomalies, thereby enhancing the quality of overall analytics.