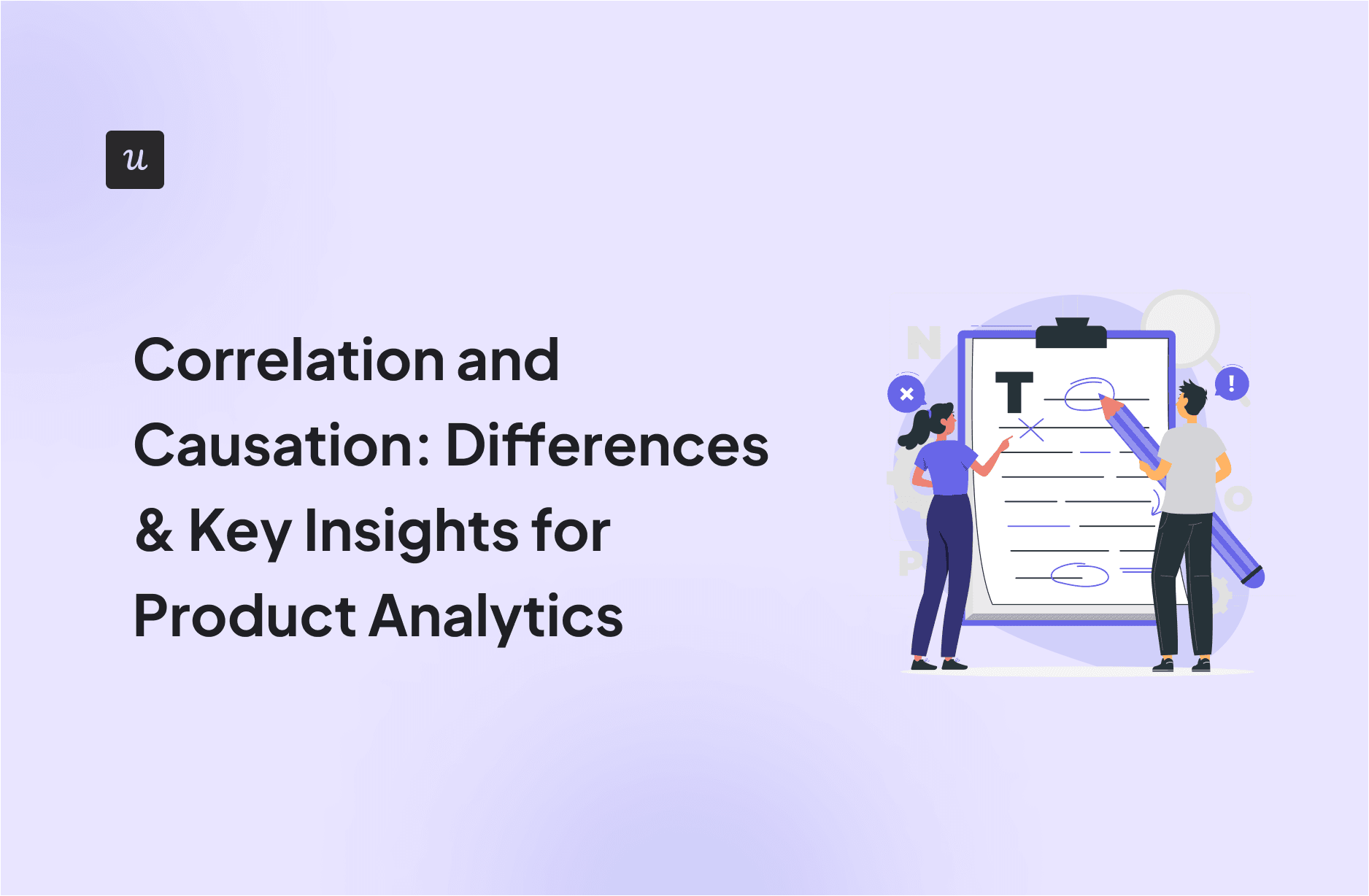
Correlation and Causation: Differences & Key Insights for Product Analytics
Have you ever questioned whether the patterns you observe in your data truly indicate one factor causing another?
This guide on ‘correlation and causation’ clarifies these concepts, essential for those seeking to make informed decisions using product analytics. We’ll also provide you with examples for a better understanding of the cause-and-effect relationship.
Get The Insights!
The fastest way to learn about Product Growth, Management & Trends.
Differences between correlation and causation
Correlation and causation are both ways of understanding relationships between variables, but they’re different concepts.
While correlation describes a relationship between variables, causation explains the direct influence one variable has on another.
For instance, in product analytics, you may notice that users who used a specific feature had higher retention than those who did not. This shows a statistical correlation, but whether there’s a causal relationship between feature usage and higher retention is still a question.
It’s also essential to note that two variables might show a correlation because of a third factor, which we call a confounding variable.
This third variable can be strongly correlated with both the variables in question, potentially misleading interpretations.
So, if you spot a correlation, don’t rush to conclusions. That’s why we say “correlation does not imply causation.” It’s a reminder to be cautious and not make snap judgments based solely on observed correlations.
Examples of correlation and causation in product analytics
Let’s get into real-life product analytics examples, where distinguishing between correlations and causations is key for valuable insights and informed decisions.
Conduct an analysis to understand user activity decline
If you see a drop activity in your product, check a Trend dashboard to track how users interact with its main features. Break it down by user plan or compare it with previous periods to find out which features might be causing the drop and who’s most affected.
Monitor feature usage patterns using Userpilot.
This analysis might show a negative correlation coefficient between feature usage and activity patterns, but it doesn’t prove what’s causing it.
For instance, you might notice that updating the onboarding process—like switching from a long product tour to a simple checklist—seems to boost product use. But to be sure, you need to run experiments and analyze the data.
That’s where methods like cohort analysis, milestone analysis, and attribution analysis come in handy. They help you figure out what actions high-value customers take and how to get more of them.
Collect data to improve your product retention
Let’s consider another scenario. To understand why users are leaving your product, you decide to implement a churn survey to capture reasons for churn and determine common causes.
Since the survey is triggered as users cancel their subscriptions, it’s contextual and can indicate the causation relationship for user churn.
This approach of data collection can help in understanding customer retention trends, which are critical for subscription-based companies. Furthermore, retention analysis enables:
- The measurement of user engagement over time.
- The comparison of various feature adoptions.
- Providing insights into which features are retaining users better.
Refine onboarding guidance to increase user activation
In another case, you notice a decline in free trial conversion. Segmenting free trial users to run a path analysis, you observe how they navigate to activation.
If they aren’t following the recommended path, this indicates a correlation, but not a statistically significant one to conclude causation.
To prove causal relationships, conducting controlled experiments and analyzing the collected data is necessary. For this, you could implement an additional survey during onboarding to capture user feedback on onboarding guidance and collect more data for conclusion.
In addition, using funnel analysis can reveal the conversion of important steps of the user journey and identify where users drop off or stay engaged.
Insights from this analysis can be invaluable in refining onboarding guidance to increase user activation.
Identify influential factors in customer satisfaction
Lastly, imagine you need to identify influential factors in customer satisfaction. Here, you analyze customer feedback across different surveys like NPS, CSAT, and CES.
For example, determining correlations between various product features and overall satisfaction levels can provide insights into which features are associated with higher satisfaction.
It’s also helpful to visualize your data using charts, such as bar charts or line charts, to understand distribution and identify the positive correlation.
However, correlation is just the first step. You may need to conduct further experimentation or qualitative research to establish causation.
Common ways to test or causation in your product
So far, we’ve highlighted the importance of understanding correlation and causation in product analytics.
Now, let’s delve into some common ways to test for causation, which can provide us with more accurate insights into user behavior and product performance.
Run A/B test experiments to identify causation
A/B testing is a method that involves comparing two versions of a web element to determine which one enhances user engagement or conversion rates. The process of A/B testing involves:
- Formulating a hypothesis.
- Determining the necessary sample size.
- Selecting treatment and control groups.
- Conducting the experiment.
- Analyzing the results.
For instance, you could evaluate two versions of in-app flows, one with a video, and one with an interactive walkthrough, to see which one drives more engagement. This testing method helps establish causation by controlling variables and measuring differences in outcomes.
Trigger contextual surveys to capture key insights
Contextual surveys are another useful tool in identifying causation relationships and providing causal evidence.
You can send these surveys after users perform a specific action to collect highly credible feedback.
For instance, you can use surveys alongside a funnel analysis to identify drop-offs in conversion. If you determine a specific step causing friction but lack data to pinpoint what the actual friction is, trigger a survey as users reach that step can help understand why users drop off.
Overall, the timing of surveys is critical; delivering them immediately after a user interaction can yield more accurate and relevant insights into the user’s motivations and perceptions.
Conclusion
In conclusion, understanding the differences between correlation and causation is not just academic jargon. It’s a crucial aspect of making informed decisions in product analytics, enabling you to drive product growth.
And if you’re looking for a great tool to help you leverage product analytics, book a Userpilot demo to see how we can help.