Types of UX Bias and How to Avoid Them
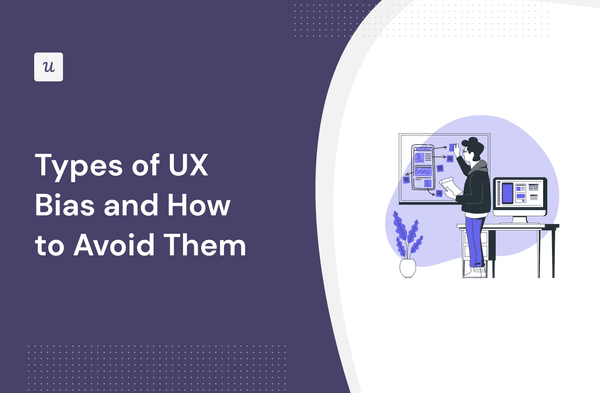
UX bias can seriously impact your research project and negatively influence your final product design. Although we can’t eliminate biases, being conscious of them helps you overcome them.
This article helps you identify potential biases affecting your UX design project. It highlights different types of biases in the user research process and how to avoid them.
TL;DR
- Cognitive biases in UX research are unconscious inclinations that impact the accuracy of your research.
- Confirmation bias is the tendency to seek out and assign value to information that confirms our already-existing beliefs.
- False consensus bias is the assumption that others think as you do and will act similarly in the same situation.
- Recency bias is the tendency to attribute greater importance to recent events than historical ones.
- Primacy bias is the tendency to remember initial events more strongly than later ones.
- Implicit bias is our unconscious stereotypes and assumptions about certain groups. It is also known as unconscious bias.
- The sunk cost fallacy is the tendency to continue with a process or project that you’ve invested time, effort, and money into, even if the costs currently outweigh the benefits.
- Social desirability bias is the tendency to respond in a way that people consider socially acceptable and preferable. This often leads to participants avoiding giving negative feedback to not upset the interviewer.
- Framing bias is the tendency to make decisions or provide responses based on how information is presented.
- The peak-end rule is the effect of assigning the greatest value to events that invoke the most intense emotions in us.
- Sampling bias involves the selection of samples that do not accurately represent the entire group.
- Anchoring bias is the tendency to rely too much on pre-existing reference points when making decisions.
- Userpilot helps you avoid biases through user segmentation and the integration of different data sources and research types. To learn more, book a demo today!
What is a cognitive bias in UX research?
Cognitive biases in UX research are unconscious inclinations, actions, and tendencies that impact the accuracy and effectiveness of your research.
Cognitive biases can influence how you prepare your study and how you interpret the data collected and the results obtained. Left unchecked, it can lead to design flaws and poor user experience.
11 Most common types of UX bias in the user research process
There are a wide variety of biases that can come to the fore when conducting research. Some of these include:
Confirmation bias
Confirmation bias refers to the tendency to search for, interpret, and assign value to information that reinforces our beliefs. It essentially involves searching for evidence to confirm what we already think.
For example, imagine you have the preconception that one gender is more creative than another. As you research the subject, you’ll easily gravitate toward “evidence” that supports your belief, while discounting other opposing findings.
When applied to UX research, confirmation bias may have you designing a product that fits your preconceived notions rather than the end user’s best interests.
False consensus bias
False consensus bias involves the assumption that others think as you do, agree with your own opinions, and will behave similarly in a given context.
In UX research, false consensus bias occurs when we assume that everyone will agree with our design or idea, without conducting research. You may even begin to believe that others with a different opinion are abnormal.
For example, you may be tasked with testing a product you’ve just worked with your company to develop. Your clear knowledge of how the product works may lead you to assume that it will be easy to use for other users.
Recency bias
This bias leads us to attribute greater importance to recent events than to older ones. Recency bias occurs in UX research when researchers assign more weight to the latest user feedback while neglecting previous ones.
For example, you may be interviewing users as part of your new product design. Towards the end of the interview, the user may mention some features they found very useful and express their love for the product.
Recency bias could lead the researcher to ignore the negative experience shared earlier in the interview by the user. This can lead them to underestimate the issue, thus, compromising the app’s overall usability.
Primacy bias
Unlike recency bias, primacy bias involves prioritizing the first piece of information encountered over any subsequent information. It involves processing information quickly rather than correctly.
For example, the first candidate in an interview may be more memorable than others that follow. Similarly, first impressions can cloud our perception of everything else a person says or does.
Actively listening and taking detailed notes during interviews can help you counter the effects of primacy bias and recency biases in UX research.
Implicit bias
Also known as unconscious bias, implicit bias refers to the stereotypes and attitudes we may associate with certain groups or individuals without our conscious knowledge.
In UX research, implicit bias could appear in our assumptions about our users. For example, UX designers may believe that their product will only be useful to a specific demographic (age, race, gender, etc.).
This may lead you to interview only users within this group, excluding other user groups as a result. Similarly, your unconscious assumptions about certain user groups may lead you to ask them potentially offensive questions while conducting interviews.
Sunk cost fallacy
The sunk cost fallacy is a common cognitive bias that arises from the financial and emotional investments we accumulate. It is the idea that the more we invest in a project, the harder it becomes to abandon it.
Put differently, the more time, effort, or resources we invest into a project or solution, the less likely we are to leave it, even when it’s not working. This is often because we don’t want to feel like we’ve wasted our time.
We see this in UX design when designers continue to work on a solution that’s not resonating with users. This may involve holding on to outdated features or iterating on a confusing original design.
Social desirability bias
This cognitive bias results from human beings’ innate desire to be part of a collective and to avoid rejection. Thus, social desirability bias occurs when people respond in a way they believe others will prefer.
For example, an interviewee may give an exaggerated amount of positive feedback on a product because they don’t want to offend the interviewer.
One way to eliminate this bias is by reinforcing confidentiality in interviews and studies. Researchers can also frame questions in a way that deflects from the interviewee to make them more comfortable with their response.
Framing bias
Framing bias occurs when a researcher frames a question in a manner that suggests a specific answer. This effect is easy to conjure as how information is presented (or worded) greatly affects our responses.
For example, asking a question such as “What did you dislike about this product” can cause the user to focus only on the negatives of the product, leading to false insights.
One way to go around this is by using neutral language. For example, you could ask, “How do you feel when using this product?” Or, “Can you describe your experience with this product?”
Peak-end rule
The peak-end rule is a phenomenon where the most intense parts of an experience (the “peak”) and the final moment in the experience (the “end”) have an outsized effect on our view of the experience.
This means that even if an overall experience is negative, our perception of the experience could be positive if it has a positive peak and a positive end.
Thus, by focusing on creating positive peaks and ends, you can design experiences that users will enjoy. For example, you can include a celebratory message after a complex task to boost user satisfaction.
Sampling bias
Sampling bias in UX research occurs when your collection of samples does not accurately represent the entire community. It involves leaving out important groups due to existing beliefs or faulty reasoning.
For instance, you may assume that your fitness product will be useful to adult males and females (ages 20-35), leaving out teenagers (ages 15-19) and seniors (ages 60+). Your product may, thus, be useful to the adults but not the others.
You can avoid sampling bias by avoiding making any assumptions. You need to first determine the full scope of your target audience before sampling representative members of that audience.
Anchoring bias
This bias occurs when we rely too much on pre-existing reference points or “anchors” when making decisions. It typically involves basing our judgments on the first piece of information we receive.
For example, highlighting your most expensive subscription plan can serve as an anchor that makes other plans look cheap.
Similarly, when researching what price users would pay for a product, the first price you mention can serve as an anchor. Asking if they’ll pay $9.99 may make them refuse to pay higher because they’ve mentally tied the product’s value to $9.99.
How to avoid user research bias as a UX designer
Biases are a natural part of the human state. Your job is to recognize your biases and those you could invoke in others, and work to eliminate them.
So, how can you eliminate bias in the UX research process? Consider five (5) helpful tips.
Collect data at multiple stages of the research and design process
One effective way to combat bias in UX research is by collecting data at multiple research and product development stages.
This means collecting data at the beginning to identify user needs and expectations and later on to evaluate how well your product satisfies those needs.
Constantly collecting, comparing, and updating data helps you maintain accuracy. It highlights biases in different data sources, strengthens your research validity, and reduces the impact of individual biases.
Recruit a representative group of participants
A key step to avoiding sampling bias is to ensure your selected sample of participants represents all user groups. Your selected research participants should properly represent the target group you are designing for.
For example, if you’re conducting a new feature research, you must first determine the user personas that will benefit from it. Use segmentation to accurately identify these users and recruit participants.

Recruiting a representative sample will ensure that your data reflects the diverse perspectives of your target audience. It prevents skewed results, helping you to make informed and impactful decisions.
Use multiple data sources when conducting user research
Relying on a single data source in user research can lead to biased findings and inaccurate conclusions. Utilizing multiple data sources, however, provides a more comprehensive view of user needs and behaviors.
Your ideal data source will depend on the stage of your research and development process. Potential sources include:
- User testing: Involves inviting real users to perform specific tasks in realistic conditions. Usability tests help you evaluate whether a product’s interface, features, and functions are ready for use.
- User surveys: A survey consists of a series of questions that help you to gather feedback on your product. Survey responses can be open- or close-ended.
- Customer service logs: These are records of interactions between a customer and your support agent – by phone, email, or in person. It is a good source of information on customer needs and challenges.
- Product analytics: Product analytics data tells you how users engage with your product or service.

For example, you may notice that your website visitors aren’t completing the checkout process. Before surveying to get their opinion, you can first dig into the analytics data.
The data may reveal that dropoffs happen later in the checkout flow after clicking the checkout button. With this information, you can ask better-tailored questions that reveal the true source of the problem.
Mix quantitative and qualitative research
Combining quantitative and qualitative data sources offers contrasting perspectives and minimizes biases.
Where quantitative research provides insight into user behavior and trends, qualitative research reveals underlying user motivations and emotions. This combination provides a clearer picture of the user experience.
You can, for example, combine qualitative and quantitative research questions in your survey. You do this by first asking close-ended questions to discern trends.

Then, you can allow users to express their independent ideas by following up with open-ended questions. This should provide deeper insight into the motivation behind each user’s response.

Restrain from leading questions to avoid confirmation bias
By their very nature, leading questions prompt and encourage interviewees or survey respondents to provide a particular answer. Such questions trigger the framing bias, leading to misleading responses.
You can avoid this by using neutral language that isn’t suggestive. For example, rather than asking, “How great was our customer service representative?” you could ask, “How would you rate the customer service representative?”
The first question assumes the experience was “great” and nudges the respondent to think the same. The second question, however, encourages independent thinking and more accurate responses.
Conclusion
Identifying bias in your research can appear tricky at first, but it doesn’t have to be. Familiarize yourself with the biases you can encounter, watch out for them, and take steps to eliminate them from your research.
To help you eliminate user research bias, Userpilot provides multiple data sources, aids data-backed user segmentation, and helps you conduct both quantitative and qualitative research. Book a demo now to learn more!