Correlation vs. Causation: Difference, Example, Process, and More
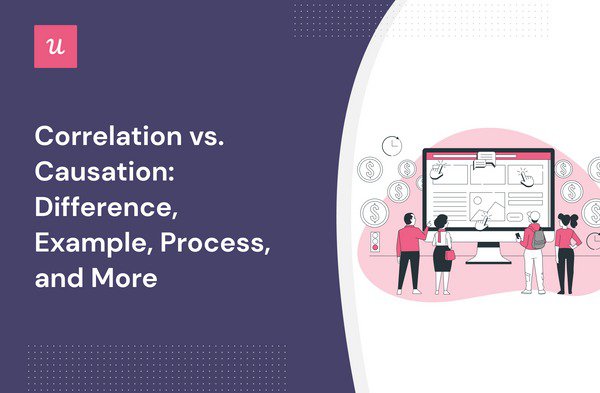
Knowing the difference between correlation vs causation can make a huge impact on the effectiveness of your product initiatives.
Our article will show you how to distinguish between the two concepts so that you can make better-informed product decisions.
Let’s get right to it!
TL;DR
- In statistics, a correlation exists when two events or values are related to each other, just like two people walking together and changing pace at the same time.
- If the correlation is positive, the values move in the same direction, and if negative – in the opposite. If there’s no relationship, it’s zero correlation.
- The correlation coefficient tells us how much the events are correlated.
- Causation is when one event causes another event. For example, improving your website copy can cause more conversions.
- Correlation doesn’t imply causation. Even when two or more variables correlate, their cause may be somewhere else.
- Distinguishing between correlation vs. causation will help you make more accurate predictions and focus your efforts on the motions that drive change.
- Determining causal relationships requires experimentation and statistical analysis.
- First, you choose your variables. An independent variable is what you think causes the change, while a dependent variable is the outcome.
- Next, you choose the experimental and control group. It’s important that participants come from the same segment and are assigned to each group randomly.
- To collect the data, you carry out an A/B test.
- Once you have the data, carry out statistical analysis to determine if the results are statistically significant and if there’s causation. It’s best to leave this bit to a data scientist.
- If there’s a true causal relationship, you should be able to replicate the results with the different data sets.
- If you want to see how Userpilot can help you test for correlation vs. causation, book the demo!
What is correlation?
Correlation is a statistical measure that shows how two variables are related to each other.
We use it to determine if there is a relationship between two variables, or in other words, how one variable changes when the other changes.
Imagine two people walking together. When both of them change pace at the same time, that’s a correlation.
What are the different types of correlation?
Correlation can be either positive or negative, indicating the direction of the relationship. We also distinguish zero correlation.
Let’s look into these types in detail:
- Positive correlation means that two variables move in the same direction. When one increases, so does the other. When one of our walkers from the example above speeds up, the other follows suit.
- Negative correlation means that the variables move in opposite directions. When one increases, the other decreases. That’s when one of our walkers slows down when the other one speeds up.
- Zero correlation is when the two variables are independent of each other and do not have any relationship. If we follow the analogy of walkers, their pace changes are random, with no relationship to each other.
Correlations are hardly ever perfect. That’s why when measuring correlation, we use the correlation coefficient. A perfect positive linear relationship has a coefficient of +1. In real life, however, this value falls somewhere between 0 and +1 (or -1 for negative correlations).
What is causation?
Causation is when a change in one variable causes a change in another variable, or one event directly causes another event.
Let’s go back to our two walkers. As they’re heading towards the zebra crossing, they can see that the light is about to change, so they start running. The change in their pace is caused by another event, the changing light.
Correlation vs. causation: What is the difference?
On the surface, correlation and causation may appear to be similar but they’re actually very different.
As mentioned, causation is when one event leads to another event. For example, your marketing team launches a new campaign that results in increased website traffic and boosts conversion rates. That’s one event causing two other events.
The increase in traffic and increase in conversion rates are correlated but there’s no causal relationship between them. They change not because of their direct interaction but because of the third variable – the brilliant marketing campaign.

Why is it important to understand the distinction between correlation and causation?
It’s easy to confuse correlation and causation.
Let’s look at the two variables above: the increased website traffic and increased website conversion rate in isolation. If you don’t know the context (the new marketing campaign), you may assume that one of them causes the other. For example, the higher website traffic causes a higher conversion rate.
What would be the consequence of acting on this assumption?
Well, if you wanted to further increase the conversion rates, you would focus your efforts on driving more traffic to the website, for example through paid ads.
However, this would increase the number of traffic because more people would be visiting the website but wouldn’t increase the conversion rate. This would, as a result, waste a lot of time and money.
So in a nutshell, determining whether you’re dealing with causation or correlation can help you make more accurate data forecasts, adopt the right strategies, and allocate resources to initiatives that have an actual impact.
What is an example of correlation and causation in product analytics?
Let’s imagine you’re a product manager of a social media management tool.
As your product has been underperforming in terms of adoption and conversions to the premium plan, you’ve decided to overhaul the onboarding process. Instead of a long product tour, you’ve created a checklist that guides new users through the features they need to realize the product value.
After a few months, you can see a hike both in product adoption and premium subscriptions among users who have completed the checklist. You may assume that the introduction of the checklists caused the boost in your key metrics.
However, all this tells you is that there might be a correlation between checklist completion and the performance of the two metrics. To determine causation, you need to conduct further tests.

How to test for causation in your SaaS?
Testing for causation can be a complex process as it requires discipline and often complex statistical operations. Let’s check out how you do it, step by step.
Define the causal relationship between two variables
You start the process by formulating a hypothesis to test and defining the independent and dependent variables.
In the checklist example, our null hypothesis would be that there’s no causal relationship between checklist completion and premium conversion, while the alternative hypothesis would be that there is a relationship between them.
In this case, the checklist completion would be the independent variable, aka the predictor. That’s the one you suspect causes the change. The premium conversion rate is the one we expect to change. We call it the dependent variable, or the outcome.

Identify the control group and treatment group
To test your hypotheses, you will need two groups. The treatment, or experimental group, is the one that will complete the checklist. The control group will not have a chance to do so.
When choosing your groups, make sure they’re as homogenous as possible. For example, choose users that hold the same role in the company, work for companies of a similar size, have the same JTBDs, or come from the same age group.
Why does it matter? Each of these factors could be a confounding variable. These are other variables that could skew the test results. To further limit the impact of confounding variables, assign users to each group randomly.
Choosing the right users for your experiment should be pretty easy with your product analytics tool as it should let you segment your users based on chosen properties.

Run experiments to determine the underlying causal relationship
Anecdotal evidence or personal observations that you may have used to formulate your hypotheses aren’t enough to determine causation or even correlation. For that, we need to carry out experiments and analyze the data we’ve collected.
Conduct A/B testing
A/B testing is a common way to collect data for hypothesis testing.
This involves exposing the treatment group to the independent variable and collecting data on how it affects the dependent variable.
So in our case, we’d enable the onboarding checklist for half of the users we’ve chosen for the test and compare how their premium conversion rates stake against those users who haven’t completed it. This is called a controlled A/B test.
Depending on your hypothesis, head-to-head tests, where you compare the impact of two independent variables, like two different checklists, a checklist and a product tour, or multivariate tests, may be more suitable.

Analyze the data for hypothesis testing
Once you have the data from the experiment, make sure to filter and organize it. For example, remove the data for users who were exposed to the checklist but dropped off at one of the steps or dismissed it altogether.
Next, you have to run a statistical test to validate your hypothesis.
The choice of the test will depend on what kind of data you’ve used and how many variables are involved. In our case, we could use a simple linear regression test to test for causality and a paired t-test to see if the results of the two groups are statistically significant.
The choice of the right test isn’t easy though and if you choose the wrong one, the results will be meaningless, so it’s best to leave this part for the data analyst on your team.
Replicate and validate the cause-and-effect relationship
Testing your hypothesis once may not be enough to determine causal relationships.
To truly validate cause-and-effect relationships between the variables, it’s necessary to carry out multiple tests on various data sets over time. If the results are replicable, you’ve nailed it.
Conclusion
The difference between causality and correlation can fundamentally impact your product decisions. Identifying the true relationships between events and metrics is essential for teams to use their resources well and prioritize initiatives to drive the desired outcomes.
If you want to see how Userpilot can help you track product usage data, select the right users for experiments, and conduct A/B tests, book the demo!