How to Conduct Qualitative Data Analysis? (+The Best Tool to Use)
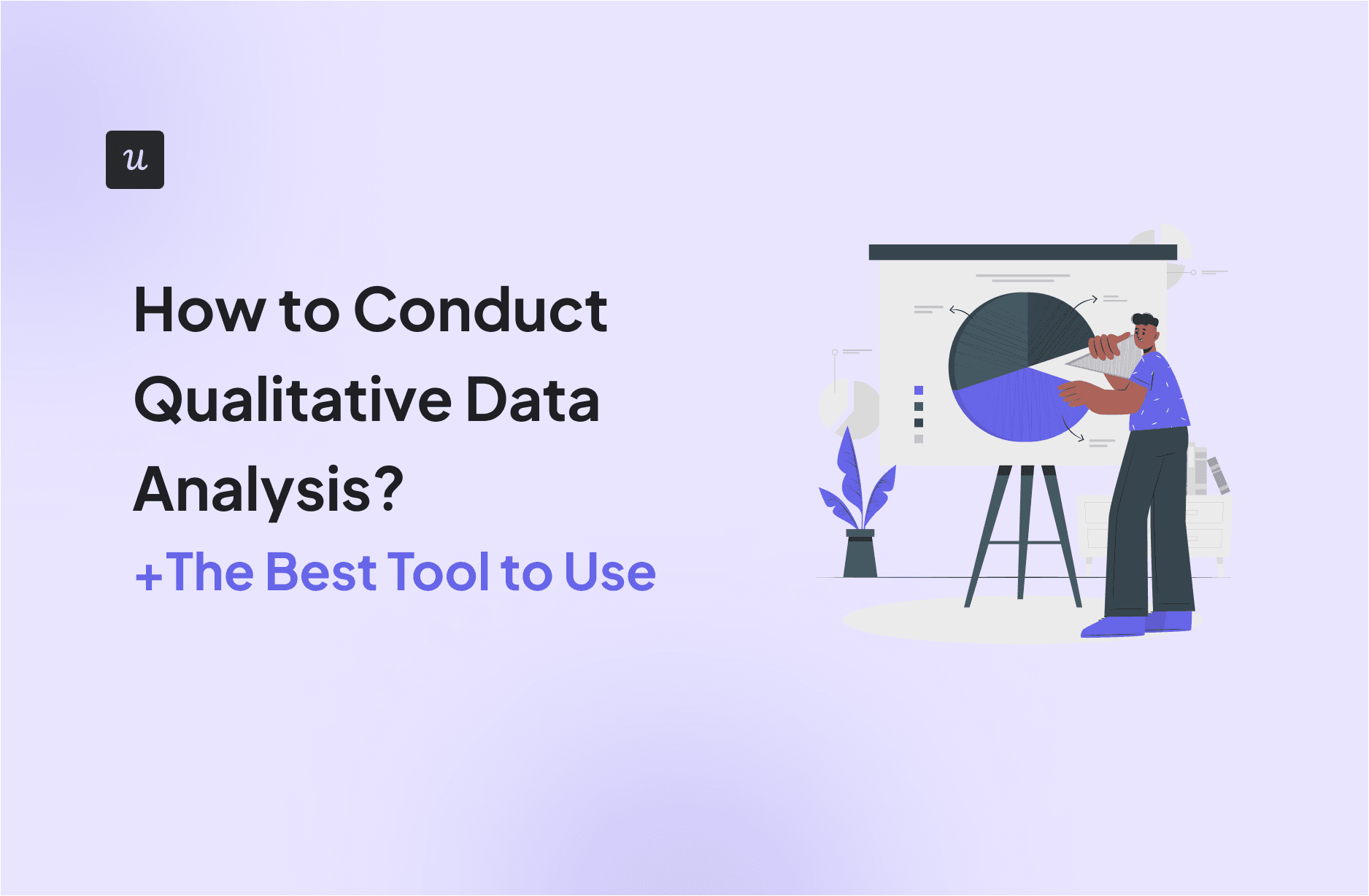
Let’s face it: qualitative data analysis is vital to understanding why users act in a particular way and how they feel about your product in a way that quantitative product analytics can’t.
This article will teach you how to analyze qualitative data to inform product development and improve the product experience.
You will discover:
- Five qualitative data analysis methods.
- A six-step analysis process and how to streamline it with Userpilot.
- How to act on your research findings.
What is qualitative data analysis?
Qualitative data analysis (QDA) involves organizing, examining, and interpreting non-numerical data collected from customers. For example, their survey responses or session recordings.
The purpose?
To understand their interactions with the product and gain in-depth insights into user pain points, unmet needs, motivations, and expectations.
Quantitative data analysis vs. qualitative data analysis
Quantitative and qualitative data analyses have different objectives and use different data types, methods, and tools to achieve them.
- The aim of quantitative research is to illustrate objectively what happens inside the product, while qualitative research focuses on the why. For example, quantitative data can reveal the percentage of users who drop off at a particular touchpoint, whereas qualitative data explains the reasons for that.
- Quantitative data analysis uses numerical and measurable data, such as event occurrences. In contrast, qualitative data analysis relies on non-numerical and descriptive data, like open-ended survey responses.
- To collect quantitative data, you use closed-ended survey questions and analytics tools to track user actions. Qualitative data, on the other hand, comes from open-ended survey questions, interviews, focus groups, phone calls and chats, and session recordings.
- Analyzing quantitative data involves visualizing it in charts and graphs and conducting statistical operations. Qualitative analysis is about finding themes and patterns in data, often manually.
Benefits of qualitative data analysis
Here are the key advantages of qualitative data analysis that underscore its importance in customer and market research:
- Deep insights: Qualitative analysis helps understand complex patterns by looking at reasons and perspectives behind the data.
- Flexibility: It allows researchers to adjust their approach as they continuously discover new information or themes.
- Contextual understanding: It explores contextual factors, which adds depth to number-based findings and reveals hidden connections.
- Participant voice: It highlights what participants actually say, experience, and feel and uses their input to shape the analysis and the results.
Challenges of qualitative data analysis
Let’s get it straight: qualitative data analysis comes with its challenges. The key ones include:
- Data overload and management: Qualitative data analysis involves tons of text or multimedia, which is challenging to organize, manage, and analyze.
- Reliability and validity: Ensuring the reliability and validity of qualitative findings is difficult because the process isn’t as standardized as quantitative analysis. Personal biases can skew the results.
- Time-intensive nature: Qualitative data analysis is resource-intensive and time-consuming. It involves iterative processes of coding, categorizing, and synthesizing data.
What are the 5 qualitative data analysis methods?
There are 5 main methods of qualitative research studies:
- Content analysis.
- Narrative analysis.
- Discourse analysis.
- Thematic analysis.
- Grounded theory analysis.
Mind you, the differences between them aren’t always clear-cut, and there is always some overlap.

1. Content analysis
Content analysis is a qualitative data analysis method that systematically evaluates a text to identify specific features or patterns. This could be anything from a customer interview transcript to survey responses, social media posts, or customer success calls.
The first step in the content analysis research method is data coding, or labeling and categorizing.
For example, if you are looking at customer feedback, you might code all mentions of “price” as “P,” all mentions of “quality” as “Q,” and so on.
Once manual coding is done, start looking for patterns and trends in the codes.
While it’s a qualitative approach, it often aims to quantify the content, for example, by counting how many times a word or theme appears.
One of the main advantages of content analysis is its relative simplicity – anyone with a good understanding of the data can do it.

The advantages of the content analysis process
- Content analysis can provide rich insights into how customers feel about your product, what their unmet needs are, and their motives.
- Once you have developed a coding system, content analysis is relatively quick and easy because it’s a systematic process.
- Content analysis requires very little investment since all you need is a good understanding of the data, and it doesn’t require any specialist qualitative research data analysis software.
The disadvantages of the content analysis process
- Coding data takes time, particularly if you have large amounts to analyze.
- Content analysis can ignore the context in which the data was collected. This may lead to misinterpretations.
- Some people argue that content analysis is a reductive approach to qualitative data because it involves breaking the data down into smaller pieces and quantifying, which can lead to oversimplifications.
2. Narrative analysis
Narrative analysis involves identifying, analyzing, and interpreting customer stories, for example, in the form of customer interviews or testimonials.
This kind of analysis helps product managers understand customers’ feelings toward the product, identify trends in customer behavior, and personalize their in-app experiences.
The advantages of narrative analysis
- The stories people tell give a deep understanding of customers’ needs and pain points.
- It collects unique, in-depth data based on customer stories.
The disadvantages of narrative analysis
- Hard to implement in large-scale studies.
- Transcribing customer interviews or testimonials is labor-intensive.
- Impossible to replicate as it relies on unique customer stories and your ability to interpret them.
3. Discourse analysis
Discourse analysis is about understanding how people communicate with each other. You can use it to analyze written or spoken language.
For instance, product teams can use discourse analysis to understand how customers talk about their products on the web.
The advantages of discourse analysis
- Uncovers emotions behind customers’ words.
- Gives insights into customer data.
The disadvantages of disclosure analysis
- Takes a lot of time and effort as the process is highly specialized and requires training and practice. There’s no “right” way to do it.
- Focuses solely on language.
4. Thematic analysis
Thematic analysis is similar to content analysis.
It also looks for patterns and themes in qualitative data and involves labeling and categorizing the data.
The difference?
It focuses on the meaning of the data and how the themes relate to the research questions.
You can pair it with sentiment analysis to determine whether a piece of writing is positive, negative, or neutral. This is done using a lexicon (i.e., a list of words and their associated sentiment scores).
SaaS companies use thematic analysis to analyze qualitative NPS survey responses to identify patterns among their customer base.
The advantages of thematic analysis
- Anyone with little training on how to label the data can perform thematic analysis.
- Survey or customer interview raw data can be easily converted into insights and quantitative data with the help of labeling.
- If done automatically, it’s an effective way to process large amounts of data (you need AI tools for this).
The disadvantages of thematic analysis
- If the data isn’t coded correctly, it can be difficult to identify themes since it’s a phrase-based method.
- It’s difficult to implement from scratch because balancing the themes and categories is tricky. They shouldn’t be too generic or too large.
5. Grounded theory analysis
Grounded theory analysis is a method used by qualitative researchers when there is little existing theory on the topic. Instead of starting with hypotheses and testing them through research, it involves generating them from the data on the fly as it emerges.
The grounded theory approach is useful for product managers who want to understand how customers interact with their products. You can also use it to generate hypotheses about how customers will behave in the future.
Suppose product teams want to understand the reasons behind the high churn rate. They can use customer surveys and grounded theory to analyze responses and develop hypotheses about why users churn and how to reengage inactive ones.
The advantages of grounded theory analysis
- It reduces bias because it doesn’t rely on assumptions.
- It’s great for analyzing poorly researched topics.
The disadvantages of grounded theory analysis
- It can come across as overly theoretical.
- It requires a lot of objectivity, creativity, and critical thinking.
Which qualitative data analysis method should you choose?
The choice of the appropriate qualitative data analysis method depends on various factors, including:
- Research question: Different qualitative methods are suitable for different research questions. For example, you can use content analysis to categorize and quantify customer feedback from support tickets to prioritize areas for improvement. Contrastingly, discourse analysis will show you how users feel about your product.
- Nature of data: Choose qualitative data analysis techniques that align with the data’s characteristics. For instance, content analysis is versatile and can be applied to various types of qualitative data, while narrative analysis focuses specifically on stories and narratives.
- Researcher expertise: Consider your own skills and expertise in qualitative analysis techniques. Some methods may require specialized training or familiarity with specific software tools. Choose a method that you feel comfortable with and confident in applying effectively.
- Research goals and resources: Evaluate your research goals, timeline, and resources available for analysis. Consider the balance between the depth of analysis and practical constraints. For example, narrative analysis is more time-consuming or resource-intensive than others.
How to perform qualitative data analysis? A step-by-step process
With all that qualitative research methods covered, it’s time for our step-by-step guide to qualitative data analysis.
Step 1: Define your qualitative research questions
It’s important to be as specific as possible with the questions, as this will guide how you collect qualitative research data and the rest of your analysis.
Examples are:
- What are the primary reasons for customer dissatisfaction with our product?
- How does X group of users feel about our new feature?
- What are our customers’ needs, and how do they vary by segment?
- How do our products fit into our customers’ lives?
- What factors influence the low usage rate of the new feature?
Step 2: Gather your qualitative customer data
Now, you decide what type of data collection to use based on previously defined goals.
Here are 5 methods to collect qualitative data for product companies:
- In-app surveys: triggered at a specific time or contextually, when the user completes an action, they allow you to reach product users (not account managers) and to collect qualitative data at scale. They should include both closed-ended and open-ended follow-up questions.
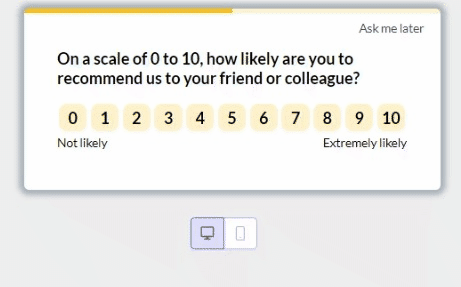
- Email surveys: delivered to users’ mailboxes, these surveys tend to have lower response rates than in-app surveys, but they’re sometimes the only way to reach inactive users.

- Review sites: Customer reviews on sites like G2, Capterra, or Clutch are often unfiltered and give you honest insights into customer sentiment and how to improve the product.

- User interviews: one-to-one conversations with customers give you the flexibility that no survey offers. A skilled interviewer can follow up on responses to help users get to the root cause of an issue, even if they’re not very good at articulating their thoughts or reflecting on their behaviors.

- Focus groups: Just like interviews, these moderated group discussions can offer valuable insights about your product and customer preferences. They aren’t very scalable, though.
Pro tip: Use a mix of in-app surveys and in-person interviews to collect qualitative data. For example, send regular NPS surveys to customers to track their sentiment over time. Segment your detractors and invite them to interviews to better understand why they’re dissatisfied with the product.
Step 3: Organize and categorize collected data
Before analyzing customer feedback and assigning any value, organize the unstructured feedback data in a single place. This is to easily detect patterns and similar themes.
One way to do this is to create a spreadsheet with all the data organized by research questions. Then, arrange the data by theme or category within each research question.
Or use Userpilot’s NPS response tagging to group similar responses. For example, you can tag all feedback about technical issues with “bug.”

Step 4: Use qualitative data coding to identify themes and patterns
Themes in data analysis help you organize and make sense of your information.
In SaaS products, common themes from customer feedback might include:
- Product issues (bugs or defects).
- Pricing concerns.
- Customer service experiences.
- Usability problems.
- User interface (UI) design.
- User experience (UX) issues.
- Missing features.
Identifying specific themes is just a start. You also need to identify their patterns: how often they occur, when, and who is affected. For example, if users complain about a missing feature, find out how many and what their JTBDs are.
You can detect those patterns using survey analytics.

Step 5: Act on the insights to improve product metrics
Once you understand the nature of the problem, look for ways to resolve it.
How you act on feedback depends on the issues.
Imagine your users request a feature. But it turns out you have a similar feature that allows them to complete the same task – they just haven’t found it. So, the solution is redesigning your onboarding process to improve feature discovery.
And if you have to build the feature, don’t just blindly follow customer requests or what competitors are doing – look for innovative solutions to get the edge.

Step 6: Continuously analyze qualitative data to stay ahead of changing customer needs
Qualitative data analysis isn’t a one-off exercise. As customer needs constantly evolve, you must always keep your hand on the pulse.
So?
Collect customer feedback in-app at regular intervals and make a habit of interviewing customers all the time, even if you’re not planning to launch new features or products.

Qualitative data analysis example: How Unolo reduced customer churn with Userpilot NPS survey
One of our customers, Unolo, faced a challenge: a high month-on-month churn rate of around 3%.
They implemented in-app NPS surveys to analyze qualitative and quantitative responses about why their customers were leaving.
In addition to collecting the feedback in-app, their customer success team also reached out to the dissatisfied customers directly.
What was the outcome?
UX and product experience improvements that reduced the churn by up to 1%!

How to perform qualitative data analysis with Userpilot?
Userpilot is a product growth platform with advanced feedback, analytics, and engagement features. So you can use it to collect qualitative and quantitative data and act on the insights to improve user experience.

Collect qualitative feedback from users with in-app surveys
You can collect qualitative user data in Userpilot through in-app surveys.
Thanks to the template library and visual editor, creating customized surveys takes minutes.
Once ready, there are two ways to trigger them.
One is by setting the date, time, and page where the user should see it. Great for regular surveys, like CSAT or NPS.
The other is through event-based triggering. That’s when you link the survey to another user action in-app, for example, using a feature. That’s how you can measure their satisfaction with specific product aspects and collect ideas on how to improve them.
In both instances, you can choose to send the survey to specific user segments.
Finally, Userpilot offers localization functionality, so you can automatically translate the survey into multiple languages for users around the globe.
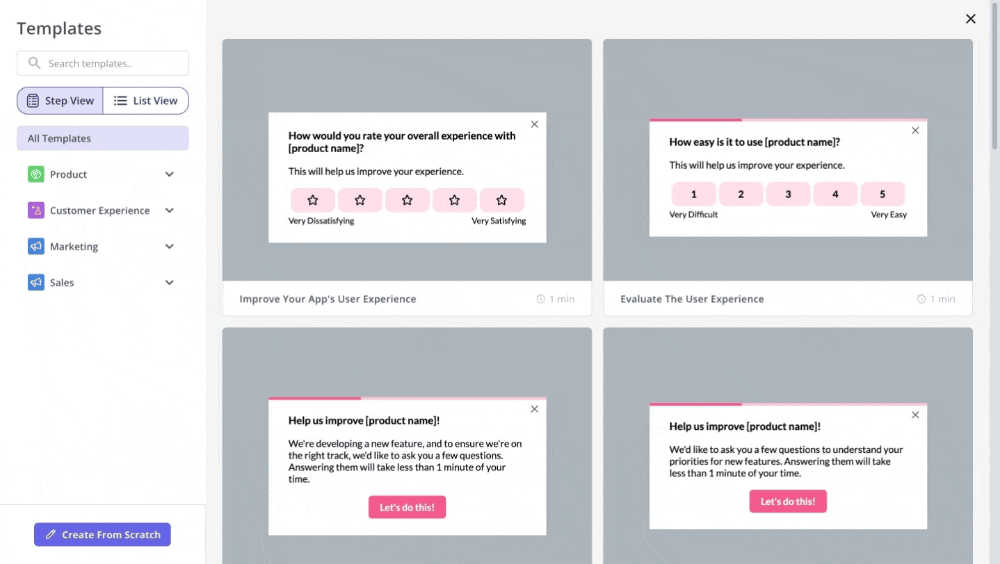
Analyze data no-code using survey analytics
Once you collect the data, you can easily analyze it without any coding.
Userpilot offers you survey analytics where you can quickly view the results and analyze user engagement with the surveys.
And let’s not forget about the NPS dashboard, which provides a comprehensive overview of your NPS scores over time, allowing you to track changes and trends in user loyalty and advocacy.
And don’t worry, Userpilot does all the NPS analysis for you: calculates the overall score and segments users into detractors, passives, and promoters.
You can also tag the NPS responses to identify common themes.

Gather customer insights via chatbots
With Userpilot, you can embed a chatbot in the resource center.
This serves two purposes:
- It allows you to support your users in-app and help them overcome issues that could lower their satisfaction and potentially lead to churn.
- You can also use it to collect qualitative customer feedback.

Build different in-app experiences based on the insights from qualitative data analysis
By analyzing qualitative feedback collected through in-app surveys, you can segment users based on these insights and create targeted in-app experiences designed to address specific user concerns or enhance key workflows.
For example, you can personalize the onboarding experiences for different user personas so that they discover the most relevant features as quickly as possible. Or use interactive walkthroughs to guide them through challenging processes.

Conclusion
The qualitative data analysis process is iterative and should be revisited as new data is collected. The goal is to constantly refine your understanding of your customer base and how they interact with your product.
Want to get started with qualitative analysis? Get a Userpilot demo and automate the qualitative data collection process. Save time on mundane work and understand your customers better!